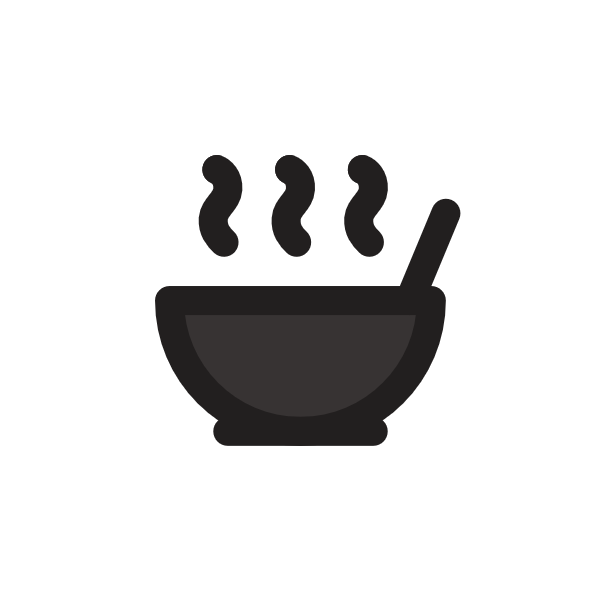
Cocojunk
🚀 Dive deep with CocoJunk – your destination for detailed, well-researched articles across science, technology, culture, and more. Explore knowledge that matters, explained in plain English.
Brain–computer interface
Read the original article here.
Brain-Computer Interfaces: Pioneering a Path Beyond Conventional Computing
Introduction: Bridging Minds and Machines – A Vision Ahead of Its Time
The concept of directly linking the human brain to external devices, bypassing the need for physical actions, might sound like science fiction. Yet, the foundational ideas of Brain-Computer Interfaces (BCIs), also known as Brain-Machine Interfaces (BMIs), were conceived decades ago, positioning them as a remarkable example of technological innovation that was significantly ahead of its time.
Brain-Computer Interface (BCI) / Brain-Machine Interface (BMI): A system that establishes a direct communication pathway between the brain's electrical activity and an external device, such as a computer or robotic limb. This interface allows for the interpretation of brain signals to control external actions or devices, effectively bypassing traditional neuromuscular pathways.
BCIs represent a paradigm shift in human-computer interaction. Instead of relying on keyboards, mice, or touchscreens, BCIs aim to translate neural signals directly into commands. This revolutionary approach holds immense potential for:
- Researching and Mapping Brain Function: BCIs offer a unique window into the brain's complex electrical activity, enabling researchers to study cognitive processes and sensory-motor functions in unprecedented detail.
- Assisting Individuals with Disabilities: BCIs can provide individuals with paralysis, amputation, or neurological disorders with new avenues for communication, mobility, and environmental control.
- Augmenting Human Capabilities: Beyond assistive applications, BCIs are explored for their potential to enhance human cognition, sensory perception, and motor skills, pushing the boundaries of human potential.
- Repairing Neurological Damage: By understanding and manipulating brain signals, BCIs could contribute to the development of therapies for neurological conditions and injuries, potentially restoring lost functions.
This educational resource delves into the fascinating world of BCIs, exploring their history, underlying principles, diverse applications, and the ethical considerations that accompany this groundbreaking technology. We will examine how the early visions of BCIs, though conceived in a time when the technology was not yet fully mature, have paved the way for today's exciting advancements and continue to inspire future innovations.
A Historical Journey: From Brainwaves to Thought Control
The journey of BCIs is deeply rooted in the understanding of the brain's electrical activity. The story begins with the pioneering work of Hans Berger in the early 20th century.
The Dawn of Electroencephalography (EEG): Unveiling Brain Rhythms
Electroencephalography (EEG): A neurophysiological measurement method to record the electrical activity of the brain using electrodes placed on the scalp. EEG is non-invasive and primarily measures voltage fluctuations resulting from ionic current flows within the neurons of the brain.
Hans Berger, a German psychiatrist, is credited with the discovery of human EEG. In 1924, he achieved the first EEG recording in humans, marking a pivotal moment in neuroscience. By analyzing EEG traces, Berger identified rhythmic patterns of brain activity, most notably the alpha wave (8–13 Hz), which is associated with a relaxed, wakeful state.
Berger's initial EEG device was rudimentary. He used silver wires inserted under the scalp, later replaced by silver foils attached to the head with rubber bandages. These sensors were connected to a Lippmann capillary electrometer, which initially yielded disappointing results. However, the introduction of more sensitive devices, such as the Siemens double-coil recording galvanometer, capable of detecting minute voltage changes (as small as 10−4 volt), led to successful and interpretable EEG recordings.
Berger's work revolutionized brain research. EEG provided a non-invasive method to study brain activity and opened new avenues for understanding brain diseases and normal brain function. His discovery laid the groundwork for future BCI development by demonstrating that brain activity could be measured and analyzed externally.
Early Conceptualizations and Artistic Explorations
While the term "brain-computer interface" was yet to be coined, the seeds of this concept were sown in the mid-20th century. One of the earliest artistic explorations of a working brain-machine interface was "Music for Solo Performer" (1965) by American composer Alvin Lucier.
Lucier's piece utilized EEG and analog signal processing hardware – filters, amplifiers, and a mixing board – to stimulate acoustic percussion instruments. The performer's brain activity, specifically the production of alpha waves, became the "controller". By generating alpha waves, the performer could "play" various percussion instruments through loudspeakers placed near or directly on them. This innovative piece demonstrated the potential of using brain signals to directly interact with and control external devices, decades before the formalization of BCI research.
The Birth of the Term "Brain-Computer Interface" and Formal Research
The 1970s marked the formal emergence of BCI research, spearheaded by Jacques Vidal at the University of California, Los Angeles (UCLA). Funded by grants from the National Science Foundation and DARPA (Defense Advanced Research Projects Agency), Vidal's work formalized the field and introduced the term "brain-computer interface" into scientific literature.
In his seminal 1973 paper, Vidal articulated the "BCI challenge"—the goal of controlling external objects using EEG signals. He specifically highlighted the Contingent Negative Variation (CNV) potential, a slow negative shift in EEG activity associated with anticipation and motor preparation, as a promising signal for BCI control.
Contingent Negative Variation (CNV): A slow, negative-going brainwave component observed in EEG recordings, typically occurring between a warning stimulus and an imperative stimulus that requires a response. It reflects anticipatory and preparatory brain activity.
Vidal's 1977 experiment provided the first practical demonstration of BCI technology. He achieved non-invasive EEG control of a cursor-like graphical object on a computer screen using Visual Evoked Potentials (VEPs). This experiment involved navigating a maze, showcasing the feasibility of controlling computer interfaces directly with brain signals.
Visual Evoked Potential (VEP): An electrical potential recorded from the visual cortex in response to visual stimulation. VEPs are used to assess visual pathway function and can be utilized in BCIs to detect responses to visual stimuli.
Expanding Horizons: From Cursor Control to Robot Manipulation
The 1980s and 1990s witnessed further advancements in BCI technology, moving beyond basic cursor control to more complex applications.
- 1988: The first demonstration of non-invasive EEG control of a physical robot was achieved. Researchers showcased EEG control over a robot's start, stop, and restart cycles of movement along a predefined trajectory on the floor. This experiment integrated autonomous robot behavior and intelligence, demonstrating the potential for brain-controlled robotics.
- 1990: A closed-loop, bidirectional, adaptive BCI controlling a computer buzzer was developed, utilizing the CNV potential. This system incorporated feedback, allowing the brain's expectation state (manifested by CNV) to control the buzzer. The resulting cognitive wave representing expectation learning was termed the Electroexpectogram (EXG), further exploring the potential of CNV for BCI applications.
Electroexpectogram (EXG): A cognitive wave pattern in EEG reflecting the brain's expectation learning, specifically related to the Contingent Negative Variation (CNV) potential.
The Neuroprosthetics Era: Restoring Lost Functions
The mid-1990s marked a significant shift as neuroprosthetic devices were first implanted in humans, following years of animal experimentation. This era saw the convergence of BCI research with neuroprosthetics, an area focused on using artificial devices to replace or restore impaired nervous system functions.
Neuroprosthetics: A field of neuroscience concerned with neural prostheses, which are artificial devices designed to replace or restore the function of impaired nervous systems, brain-related functions, or sensory and motor organs.
The first neuroprosthetic device, the pacemaker, predates modern BCI research but serves as an early example of using technology to interface with and regulate biological functions. Cochlear implants, retinal implants, and other neuroprosthetic devices followed, demonstrating the growing potential of technology to address sensory and motor impairments.
While the terms BCI and neuroprosthetics are sometimes used interchangeably, they share common goals: restoring sight, hearing, movement, communication, and even cognitive function. Both fields rely on similar experimental methods and surgical techniques, highlighting the close relationship between them.
Continued Momentum and DARPA's BRAIN Initiative
The 2010s saw continued momentum in BCI research, fueled by advancements in neural stimulation and funding initiatives like DARPA's BRAIN Initiative (Brain Research through Advancing Innovative Neurotechnologies), launched in 2013. The BRAIN Initiative significantly boosted BCI research by supporting teams at institutions like the University of Pittsburgh Medical Center, Paradromics, Brown University, and Synchron, accelerating the development of more sophisticated and clinically relevant BCI technologies.
Neuroprosthetics: Restoring Function and Enhancing Lives
Neuroprosthetics represents a significant application area for BCI technology, aiming to bridge the gap between neural signals and artificial devices to restore lost functions. While BCIs encompass a broader range of applications, neuroprosthetics focuses specifically on creating neural prostheses to address impairments in the nervous system.
As of 2010, cochlear implants stood as the most widely implanted neuroprosthetic device, benefiting over 736,900 individuals worldwide with hearing loss. Retinal implants are another prominent example, striving to restore vision in individuals with certain types of blindness.
The pacemaker, although not directly interfacing with the brain, is considered the first neuroprosthetic device, highlighting the long history of technological interventions in the nervous system.
Neuroprosthetics and BCIs share the overarching objective of improving quality of life by restoring sensory, motor, communication, and cognitive functions. They employ similar research methodologies, surgical approaches, and technological tools, demonstrating a strong synergy between these fields in their pursuit of enhancing human capabilities and addressing neurological impairments.
Animal Research: Paving the Way for Human BCIs
Animal research has been instrumental in the development and refinement of BCI technology. Studies involving monkeys and rats have demonstrated the feasibility of reading brain signals to control external devices, providing crucial insights and proof-of-concept for human applications.
Early Breakthroughs in Animal BCI Control
- 1969: Operant conditioning studies by Fetz et al. showed that monkeys could learn to control a biofeedback arm's deflection using neural activity. This early work demonstrated the brain's ability to learn and adapt to control external devices through neural signals.
- 1970s: Further studies confirmed that monkeys could learn to control the firing rates of individual and multiple neurons in the motor cortex when rewarded. This research provided deeper understanding of the neural mechanisms underlying motor control and paved the way for more sophisticated movement-based BCIs.
Decoding Movement: Algorithms and Neural Ensembles
Algorithms to reconstruct movements from motor cortex neurons have been under development since the 1970s.
- 1980s: Georgopoulos at Johns Hopkins University discovered a mathematical relationship between the electrical activity of single motor cortex neurons in monkeys and the direction of their arm movements. He also found that motor commands were collectively controlled by dispersed groups of neurons across different brain areas. This finding highlighted the complexity of neural motor control and the importance of considering neuronal ensembles in BCI design.
Several research groups have successfully captured complex brain motor cortex signals by recording from neural ensembles (groups of neurons). These signals have been used to control external devices, demonstrating increasingly sophisticated levels of BCI control.
Key Researchers and Their Contributions
- Kennedy and Yang Dan: Phillip Kennedy, founder of Neural Signals, and his colleagues developed the first intracortical brain-computer interface using neurotrophic-cone electrodes implanted in monkeys. In 1999, Yang Dan et al. at UC Berkeley achieved a groundbreaking feat by decoding neuronal firings to reconstruct images from cats' visual experiences. They used an array of electrodes in the thalamus, targeting the lateral geniculate nucleus (LGN), which processes retinal signals. By recording neuron activity while cats watched short movies, researchers used mathematical filters to decode the signals and reconstruct recognizable scenes and moving objects.
- Nicolelis: Miguel Nicolelis at Duke University advocates for using multiple electrodes spread across larger brain areas to capture neuronal signals. His research, starting with rats in the 1990s, progressed to owl monkeys, where BCIs were developed to decode brain activity and reproduce monkey movements in robotic arms. By 2000, Nicolelis's team built a real-time BCI that could reproduce owl monkey movements while they used a joystick or reached for food, even controlling a remote robot. Later experiments with rhesus monkeys incorporated feedback and successfully reproduced reaching and grasping movements in a robot arm, further refining the sophistication of BCI control.
- Donoghue, Schwartz, and Andersen: Researchers like John Donoghue at Brown University, Andrew Schwartz at the University of Pittsburgh, and Richard Andersen at Caltech have also made significant contributions, developing BCIs and algorithms to decode neuron signals. Notably, they achieved working BCIs using fewer neurons (15–30) than Nicolelis (50–200), demonstrating the potential for efficient signal decoding with smaller neuronal ensembles. These groups have trained monkeys to use BCIs for tasks like tracking visual targets, controlling robotic arms in virtual reality, and even self-feeding with brain-controlled robotic arms. Andersen's group focused on premovement activity in the posterior parietal cortex, including reward anticipation signals, expanding the range of brain signals utilized for BCI control.
Expanding BCI Applications in Animal Models
Beyond movement control, BCI research in animals has explored:
- Predicting muscle activity: BCIs are being developed to predict electromyographic (EMG) activity of primate muscles, potentially enabling paralyzed limb mobility through electrical muscle stimulation.
- Decoding arm position: Nicolelis and colleagues demonstrated that large neuronal ensembles could accurately predict arm position, allowing BCIs to translate movement intentions into actuator movements.
- Robotic appendage representation: Carmena and Lebedev and their teams showed that brain networks reorganize to represent robotic appendages as extensions of the body, alongside the animal's own limbs, highlighting the brain's remarkable plasticity.
- Speech Synthesis: In 2019 and 2021, studies reported BCIs capable of decoding neural activity related to speech, potentially aiding patients with speech impairments.
Challenges and Future Directions in Animal BCI Research
Despite significant progress, animal BCI research faces ongoing challenges. A major impediment is the need for better sensors that provide safe, accurate, and long-term access to brain signals. Improved sensor technology would expand the communication functions achievable through BCIs.
Developing and implementing BCI systems remains complex and time-consuming. To address this, Gerwin Schalk developed BCI2000, a general-purpose system for BCI research, since 2000, aiming to streamline BCI development and standardization.
Emerging technologies like "wireless" approaches using light-gated ion channels (channelrhodopsin) offer new avenues for controlling neuron activity. These techniques, demonstrated in mice, could lead to more targeted and less invasive BCI methods.
Animal BCI research has also contributed to fundamental neuroscience discoveries, including:
- Single neuron insufficiency principle: Demonstrating that single neurons, even with well-tuned firing rates, carry limited information, emphasizing the importance of ensemble recordings for high accuracy.
- Neuronal multitasking principle, neuronal mass principle, neural degeneracy principle, and plasticity principle: These principles, discovered through BCI research, have deepened our understanding of neural network function and brain organization.
Passive BCIs and User State Adaptation
Beyond active control, passive BCIs are being explored for users without disabilities. These systems assess and interpret changes in user state during human-computer interaction (HCI). In a secondary, implicit control loop, the system adapts to the user's state, improving usability and creating more intuitive and responsive interfaces.
Passive Brain-Computer Interface (BCI): A BCI system that infers a user's cognitive state or affective state without requiring conscious effort or intent from the user. Passive BCIs monitor brain activity to adapt the system to the user's needs and preferences, enhancing human-computer interaction.
Sensory BCIs and Closed-Loop Stimulation
BCI systems are also being developed to encode signals from the periphery, creating sensory BCIs. These devices enable real-time, behaviorally relevant decisions based on closed-loop neural stimulation, potentially restoring sensory feedback and enhancing interaction with the environment.
The BCI Research Award: Recognizing Innovation
The BCI Research Award is an annual recognition of innovative research in the field, highlighting the continuous advancements and breakthroughs in BCI technology. Each year, a renowned research laboratory judges projects and selects winners, further promoting excellence and innovation in the BCI community.
Human Research: Translating BCI Potential to Human Applications
Building on animal research, human studies are crucial for translating BCI technology into practical applications that can benefit individuals with disabilities and enhance human capabilities. Human BCI research encompasses various approaches, categorized by their level of invasiveness.
Invasive BCIs: Direct Access for High-Fidelity Signals
Invasive Brain-Computer Interface (BCI): A type of BCI that requires surgical implantation of electrodes directly into the brain, typically within the grey matter. Invasive BCIs offer the highest signal quality and spatial resolution but carry risks associated with surgery and potential long-term biocompatibility issues.
Invasive BCIs require neurosurgery to implant electrodes directly into the brain, under the scalp. The primary advantage of this approach is the ability to record high-quality, high-resolution brain signals directly from the grey matter. This direct access significantly enhances accuracy and control. However, invasive BCIs come with downsides, including surgical risks, potential side effects like scar tissue formation that can obstruct signals, and the risk of the body rejecting the implanted electrodes.
Vision Restoration with Invasive BCIs
Invasive BCI research has focused on restoring vision in individuals with acquired blindness (non-congenital) and providing new functionalities for people with paralysis.
William Dobelle, a private researcher, pioneered early work in vision restoration using invasive BCIs.
- 1978: Dobelle implanted his first prototype BCI in "Jerry," a man blinded in adulthood. A 68-electrode array was implanted onto Jerry's visual cortex, successfully producing phosphenes, the sensation of seeing light. The system included cameras mounted on glasses to transmit signals to the implant. Initially, Jerry could perceive shades of grey in a limited visual field at a low frame rate, requiring connection to a mainframe computer. Over time, advancements in electronics made the system more portable, enabling Jerry to perform simple tasks unassisted.
Phosphenes: The sensation of seeing light flashes or patterns in the absence of actual light entering the eye. Phosphenes can be induced by electrical or magnetic stimulation of the visual cortex or retina.
- 2002: Jens Naumann became the first of 16 paying patients to receive Dobelle's second-generation implant, marking one of the earliest commercial applications of BCIs. This improved device used a more sophisticated implant for better mapping of phosphenes into coherent vision, creating a "starry-night effect" across the visual field. Shortly after implantation, Naumann could use his restored vision to drive a car slowly in a parking area. However, after Dobelle's death in 2004, his work was not continued. Naumann and other patients experienced vision deterioration and eventually lost their "sight" again, highlighting the challenges of long-term maintenance and sustainability in early BCI implementations.
Movement Restoration with Invasive BCIs
BCIs for motor neuroprosthetics aim to restore movement in individuals with paralysis or provide assistive devices like computer interfaces or robot arms.
- Kennedy and Bakay (1998): Pioneered human brain implants that produced signals of sufficient quality to simulate movement. Their patient, Johnny Ray, who had locked-in syndrome after a brainstem stroke, received an implant in 1998. Ray learned to control a computer cursor before his death in 2002.
- Matt Nagle (2005): A tetraplegic patient, became the first person to control an artificial hand using a BCI as part of the BrainGate trial. A 96-electrode implant in Nagle's motor cortex allowed him to control a robotic arm, computer cursor, lights, and TV simply by thinking about moving his hand.
- Jonathan Wolpaw (2006): Received the Altran Foundation for Innovation prize for developing a BCI using electrodes on the scalp surface (non-invasive), offering a less invasive alternative to implanted electrodes.
- BrainGate and University of Pittsburgh Medical Center (UPMC) Teams: Demonstrated control of prosthetic limbs with multiple degrees of freedom using direct connections to neuron arrays in the motor cortex of tetraplegic patients in collaboration with the US Department of Veterans Affairs (VA).
Communication Restoration with Invasive BCIs
Invasive BCIs are also being used to restore communication for individuals with severe paralysis or speech impairments.
- Stanford University Team (2021): Reported a successful proof-of-concept test enabling a quadriplegic participant to produce English sentences at 86 characters per minute and 18 words per minute. The participant imagined handwriting, and the system used handwriting recognition on motor cortex signals using Hidden Markov models and recurrent neural networks.
- 2021 Study: Demonstrated that a paralyzed patient could communicate 15 words per minute using a brain implant analyzing vocal tract motor neurons.
- 2023 Studies: Reported record speech decoding rates of 62 and 78 words per minute using BCIs with recurrent neural networks.
These advancements suggest that BCIs could potentially surpass the information transfer rates of natural language, raising questions about the limits of human communication and the brain's information processing capacity.
Technical Challenges of Invasive BCIs
Invasive BCIs face several technical challenges:
- Signal Acquisition: Recording high-quality brain signals with implanted electrodes is technically demanding. Advances in CMOS technology are driving the development of smaller, lower-power, and higher-signal-acquisition integrated invasive BCIs.
- Electrode-Tissue Interface: Invasive BCIs rely on electrodes penetrating brain tissue to record action potential signals (spikes). The interface between the electrode and the surrounding electrolytic solution is complex and modeled using the Hodgkin-Huxley model.
- Signal Amplitude: Extracellular voltages recorded by chronic invasive BCIs are significantly smaller (microvolts) than intracellular action potential voltages (millivolts).
- Electrode Capacitance: The electrode-tissue interface exhibits high capacitance at small voltages, further complicating signal detection.
- Signal Processing and Sampling Rate: Each electrode requires its own amplifier and Analog-to-Digital Converter (ADC) to process weak analog signals. Sampling rates of 300 Hz to 5 kHz are needed to capture neuron spikes, which last approximately one millisecond.
- Power Consumption and Heat Dissipation: Invasive BCIs must be low-power to minimize heat dissipation to surrounding tissue, requiring optimized battery design.
- Material Science and Biocompatibility: Maintaining long-term signal stability is crucial. Signal quality degradation over time is a common issue, potentially due to glial scarring at the electrode-tissue interface. Research suggests that blood-brain barrier leakage contributes to inflammation and glial reaction. Flexible, tissue-like electrode designs are being explored to minimize foreign-body reactions.
Partially Invasive BCIs: Balancing Signal Quality and Invasiveness
Partially Invasive Brain-Computer Interface (BCI): A type of BCI where devices are implanted inside the skull but positioned outside the brain tissue itself, often on the surface of the brain or within blood vessels. Partially invasive BCIs offer a compromise between the high signal quality of invasive BCIs and the lower risk and complexity of non-invasive BCIs.
Partially invasive BCIs are implanted inside the skull but rest outside the brain parenchyma (functional tissue). They offer higher resolution signals than non-invasive BCIs because they bypass the signal distortion caused by the skull. They also carry a lower risk of scar tissue formation compared to fully invasive BCIs. Research has shown preclinical success with intracortical BCIs in stroke perilesional cortex (tissue surrounding the stroke area).
Endovascular BCIs: Accessing the Brain via Blood Vessels
Endovascular Brain-Computer Interface (BCI): A minimally invasive type of BCI that is inserted into blood vessels, typically veins, to reach areas near the brain. Endovascular BCIs avoid open brain surgery and its associated risks, offering a less invasive approach to accessing brain signals.
Endovascular BCIs are inserted through the vascular system, offering a less invasive surgical approach.
- Stentrode (University of Melbourne): Developed by Australian neurologist Thomas Oxley, Stentrode is a stent electrode array inserted via an intravenous catheter into the superior sagittal sinus, near the motor cortex. It measures neural activity and transmits it wirelessly to a telemetry unit implanted in the chest. The insertion procedure is similar to placing venous sinus stents. While avoiding craniotomy (opening the skull), endovascular BCIs carry risks of clotting and venous thrombosis. Human trials of Stentrode began in 2021. In 2020, two ALS patients successfully used Stentrode to wirelessly control computers for texting, emailing, shopping, and banking, marking the first human implantation of a BCI via blood vessels. In 2023, reports indicated no serious adverse events in the first year for four patients using Stentrode to operate computers.
Electrocorticography (ECoG): Recording from the Brain Surface
Electrocorticography (ECoG): A partially invasive BCI technique that measures brain electrical activity using electrodes placed directly on the surface of the brain, beneath the skull but outside the brain tissue. ECoG offers a higher signal quality and spatial resolution than EEG while being less invasive than intracortical implants.
Electrocorticography (ECoG) records brain electrical activity from beneath the skull, similar to EEG, but using electrodes embedded in a thin plastic pad placed on the cortex, beneath the dura mater (outermost membrane covering the brain).
- 2004: Eric Leuthardt and Daniel Moran at Washington University in St. Louis pioneered human trials of ECoG technologies. Later trials enabled a teenager to play Space Invaders, demonstrating rapid control with minimal training.
ECoG signals can be subdural (beneath the dura mater) or epidural (above the dura mater) but are not taken from within the brain parenchyma. ECoG is often used in patients undergoing invasive monitoring for epilepsy treatment.
ECoG offers advantages over both EEG and invasive BCIs:
- Higher spatial resolution and signal-to-noise ratio than EEG.
- Wider frequency range than EEG.
- Less training required than EEG.
- Lower technical difficulty and clinical risk than invasive BCIs.
- Potentially superior long-term stability compared to intracortical recordings.
These features make ECoG promising for real-world applications for individuals with motor disabilities. Edward Chang and Joseph Makin at UCSF demonstrated that ECoG signals could decode speech from epilepsy patients with high accuracy using encoder-decoder neural networks, achieving word error rates as low as 3%.
Functional Near-Infrared Spectroscopy (fNIRS): Non-Electrical Brain Monitoring
Functional Near-Infrared Spectroscopy (fNIRS): A neuroimaging technique that measures brain activity by detecting changes in blood flow using near-infrared light. fNIRS is a non-invasive and portable method that can be used in BCI applications to detect cognitive and motor states.
Functional near-infrared spectroscopy (fNIRS) uses near-infrared light to measure brain activity by detecting changes in blood flow. In 2014, an fNIRS-based BCI restored basic communication for "locked-in" ALS patients, demonstrating its potential for individuals with severe communication impairments.
Non-Invasive BCIs: Accessible and Safe Brain Signal Acquisition
Non-Invasive Brain-Computer Interface (BCI): A type of BCI that records brain activity from outside the skull, typically using electrodes placed on the scalp. The most common non-invasive BCI method is electroencephalography (EEG). Non-invasive BCIs are safer and easier to use than invasive BCIs, but they generally have lower signal quality and spatial resolution.
Non-invasive BCIs are the least invasive approach, using sensors placed on the scalp to record brain activity. Electroencephalography (EEG) is the most common non-invasive BCI method. While less invasive and safer, non-invasive BCIs typically have lower signal quality and spatial resolution compared to invasive or partially invasive methods, as the skull bone and scalp tissue distort and attenuate brain signals.
EEG-Based BCIs: A Versatile Non-Invasive Approach
After Vidal's initial BCI challenge, early non-invasive BCI reports included:
- Cursor control in 2D using VEPs.
- Buzzer control using CNV.
- Physical robot control using alpha brain rhythms.
- Text writing on screen using P300 potentials.
Challenges of Early EEG-Based BCIs:
- Extensive Training: Early EEG-based BCIs often required lengthy and intensive training for users to achieve control. For example, Niels Birbaumer's research in the mid-1990s involved training paralyzed individuals for months to self-regulate slow cortical potentials for binary cursor control, which was slow and laborious.
- Slow Cortical Potential Approach: This early approach, while pioneering, has largely been replaced by faster, more accurate, and less training-intensive methods.
Advancements in EEG-Based BCIs:
- Oscillatory Activity and User Choice: Researchers like Gert Pfurtscheller, Birbaumer, and Jonathan Wolpaw focused on developing technology that allowed users to choose the brain signals easiest for them to control a BCI, including mu and beta rhythms.
- Feedback Methods and P300 Signals: P300 waves, generated involuntarily upon recognizing a stimulus, offer a feedback mechanism that requires less training. P300-based BCIs can decode categories of thoughts without extensive user training.
- EEG Emulation of Digital Circuits: Studies have demonstrated EEG emulation of digital circuits like flip-flops, Schmitt triggers, demultiplexers, and modems, showcasing the potential for complex control using EEG signals.
- Advanced Neuroimaging and Source Imaging: Bin He and his team at the University of Minnesota have advanced EEG-based BCIs using functional neuroimaging (fMRI) and EEG source imaging. They identified the co-variation and co-localization of electrophysiological and hemodynamic signals. By refining neuroimaging approaches and training protocols, they developed non-invasive EEG-based BCIs to control a virtual helicopter in 3D space based on motor imagery. They also addressed the EEG inverse problem, using virtual EEG for BCI tasks, demonstrating the merits of source analysis-based BCIs.
- Improved Communication for Motor-Impaired Patients: Studies have shown that non-invasive EEG BCIs can enable faster and more reliable communication for severely motor-impaired patients compared to muscle-based communication methods.
- Evolutionary Algorithms and Consumer-Grade Devices: Evolutionary algorithms have been applied to improve EEG mental state classification using consumer-grade devices like the Muse headband, broadening access to BCI technology.
- Post-Stroke Rehabilitation: Systematic reviews have reported the efficacy of EEG-based BCIs in improving upper-limb motor function in post-stroke rehabilitation, particularly when using band power features, motor imagery, and functional electrical stimulation. Robot-assisted EEG-based BCI has also shown promise for hand rehabilitation after stroke.
Dry Active Electrode Arrays: Simplifying EEG Acquisition
Dry Active Electrode Arrays: A type of EEG electrode that does not require conductive gel or skin preparation for signal acquisition. Dry electrodes offer advantages in terms of convenience, ease of use, and reduced skin irritation, making EEG more accessible for BCI applications.
In the early 1990s, Babak Taheri at UC Davis developed the first single and multichannel dry active electrode arrays. These arrays, with integrated electronics for noise reduction, offered advantages over traditional silver/silver chloride electrodes:
- No electrolyte gel needed.
- No skin preparation required.
- Significantly reduced sensor size.
- Compatibility with EEG monitoring systems.
These active electrode arrays are integrated systems containing capacitive sensors with local circuitry and batteries. They have been successfully tested in various EEG modalities, including spontaneous EEG, event-related potentials, brain stem potentials, and cognitive event-related potentials, showing comparable or superior performance to wet electrodes with improved ease of use and signal quality.
SSVEP Mobile EEG BCIs: Wearable and Real-Time Control
Steady-State Visually Evoked Potential (SSVEP): An electrical brain response elicited by flickering visual stimuli at specific frequencies. SSVEPs are robust and easily detectable in EEG signals, making them suitable for BCI applications, particularly for mobile and real-time control systems.
Steady-state visually evoked potential (SSVEP) mobile EEG BCIs utilize visual stimuli to elicit brain responses detectable in EEG signals.
- NCTU Brain-Computer-Interface-headband (2009): Announced a headband with silicon-based MEMS dry electrodes for non-hairy body sites. This system measured alpha activity and transmitted it via Bluetooth to a phone to assess alertness and cognitive capacity, providing feedback to rouse drowsy users.
- Cellular-Based BCI (2011): Researchers reported a wearable cellular BCI using SSVEPs that could trigger phone calls. Participants could initiate calls with minimal practice in natural environments, demonstrating the feasibility of mobile and user-friendly BCI systems.
- Mobile BCI Algorithm Development: Research has focused on developing algorithms like single-channel Fast Fourier Transform (FFT) and multiple-channel Canonical Correlation Analysis (CCA) to support mobile BCIs with high accuracy and speed. CCA algorithms have shown robustness to motion artifacts, crucial for mobile applications.
- Mobile Platform Testing (2013): Comparative tests on Android phones, tablets, and computers analyzed EEG SSVEP power spectrum density, aiming to improve the practicability and portability of SSVEP-based BCIs for daily use. While phones showed less stable signals, the feasibility of mobile stimulus BCIs was demonstrated.
- Motion Artifact Mitigation: Studies have investigated motion artifacts in mobile EEG-based BCIs. Independent Component Analysis (ICA) has been shown to be effective in separating EEG signals from noise in mobile settings.
Limitations of EEG-Based BCIs and Potential Solutions:
- Low Spatial Resolution: EEG has limited spatial resolution. Solutions being explored include EEG source connectivity analysis based on graph theory, EEG pattern recognition using Topomaps, and EEG-fMRI fusion to combine the strengths of both modalities.
Prosthesis and Environment Control with Non-Invasive BCIs
Non-invasive BCIs have been successfully applied to control prosthetic upper and lower extremity devices.
- Pfurtscheller et al.: Demonstrated a BCI-controlled functional electrical stimulation system to restore upper extremity movements in a tetraplegic individual.
- University of California, Irvine (2012-2013): Showed that BCI technology could restore brain-controlled walking after spinal cord injury using a BCI-robotic gait orthosis.
- Alex Blainey (2009): An independent researcher, used the Emotiv EPOC to control a 5-axis robot arm, demonstrating mind-controlled wheelchairs and home automation systems, showcasing the potential for non-invasive BCIs in everyday applications.
Magnetoencephalography (MEG) and Functional MRI (fMRI) as Non-Invasive BCIs
Magnetoencephalography (MEG): A neuroimaging technique that measures magnetic fields produced by electrical currents in the brain. MEG offers high temporal resolution similar to EEG but with better spatial resolution and is non-invasive. Functional Magnetic Resonance Imaging (fMRI): A neuroimaging technique that measures brain activity by detecting changes in blood flow, which is correlated with neural activity. fMRI provides high spatial resolution but has lower temporal resolution compared to EEG and MEG.
Magnetoencephalography (MEG) and functional magnetic resonance imaging (fMRI) have also been explored as non-invasive BCI modalities.
- fMRI Pong Game: fMRI was used to enable two users to play Pong in real-time by modulating their haemodynamic response (brain blood flow) through biofeedback.
- fMRI Robot Arm Control: Real-time fMRI measurements of haemodynamic responses have controlled robot arms, albeit with a seven-second delay between thought and movement due to the slower haemodynamic response.
- fMRI Image Reconstruction: ATR Computational Neuroscience Laboratories in Kyoto, Japan (2008): Reconstructed images from brain signals using fMRI at a resolution of 10x10 pixels.
- fMRI Video Reconstruction (2011): Researchers achieved second-by-second video reconstruction from fMRI data by creating a statistical model relating videos to brain activity and matching brain activity patterns to a database of video segments.
BCI Control Strategies in Neurogaming
BCIs are increasingly explored for neurogaming applications, offering novel and immersive gaming experiences. Different BCI control strategies are utilized in neurogaming:
- Motor Imagery: Involves imagining body movements to activate the sensorimotor cortex, modulating EEG sensorimotor oscillations. BCIs detect these oscillations to infer user intent. Motor imagery typically requires training, which can be time-consuming, and control can be slow. Machine learning methods, like Filter Bank Common Spatial Pattern (FBCSP), are used to improve motor imagery detection.
- Bio/Neurofeedback for Passive BCIs: Biofeedback monitors mental relaxation and physiological parameters like EMG, GSR, and HRV. EEG biofeedback systems monitor brainwave bands (theta, alpha, SMR, beta) and train users to control them. Passive BCIs use biofeedback to enrich human-machine interaction by adapting to user mental states, enhancing game experiences by responding to player cognitive states without explicit control commands.
- Visual Evoked Potentials (VEPs): VEPs, including SSVEPs and P300 potentials, are elicited by visual stimuli.
- SSVEPs: Robust signals generated by flickering visual stimuli, easily detectable in EEG. SSVEP-based BCIs require users to gaze at flashing symbols, which can become irritating during long sessions.
- P300 Potentials: Positive EEG peaks occurring after target or oddball stimuli. P300-based BCIs require less training and offer discrete selections. The P300 matrix, where users select letters from a grid by focusing on flashing rows and columns, was an early application. P300 offers slower communication rates but simplifies gameplay by requiring minimal user training.
Non-Brain-Based Human-Computer Interfaces (Physiological Computing)
Human-computer interaction extends beyond BCIs to include other physiological recording modalities, such as electrooculography (EOG) and eye-tracking, which do not directly measure brain activity but can be used for interface control.
- Electrooculography (EOG): Measures eye movements. In 1989, EOG signals were used to control a mobile robot with commands like forward, backward, left, right, and stop.
- Pupil-Size Oscillation: A non-EEG-based HCI based on covert interest and pupil-size oscillation. Users direct attention to a letter on a virtual keyboard without direct gaze, and letter selection is based on matching pupil-size oscillations to background circle brightness oscillations. Mental rehearsal enhances accuracy.
Brain-to-Brain Communication: Interconnecting Minds
Brain-to-brain communication represents an even more futuristic application of BCI technology, exploring the direct exchange of information between brains.
- Early Morse Code Communication (1960s): A researcher trained to create Morse code using alpha waves through EEG.
- Rat Brain-to-Brain Interface (2013): Miguel Nicolelis's group connected the brains of two rats, enabling information sharing, marking the first direct brain-to-brain interface.
- ECoG Speech Discrimination: Gerwin Schalk showed that ECoG signals could discriminate vowels and consonants in spoken and imagined words, providing a basis for brain-based communication using imagined speech.
- Human Nervous System Internet Link (2002): Kevin Warwick implanted 100 electrodes into his nervous system to link it to the internet and conducted experiments, including direct electronic communication between his and his wife's nervous systems (also implanted).
- Non-Invasive Human Brain-to-Brain Communication: Researchers achieved brain-to-brain communication between distant participants using non-invasive scalp technology. Words encoded in binary streams were transmitted mind-to-mind, demonstrating the potential for non-invasive brain-to-brain communication.
Cell-Culture BCIs: Interfacing with Neural Networks In Vitro
Cell-Culture Brain-Computer Interface (BCI): A BCI system that interfaces with neural cells and neural networks grown in vitro (outside the body), typically on microelectrode arrays. Cell-culture BCIs are used to study neural network function, computation, and problem-solving, and for manipulating robotic devices in a controlled laboratory setting.
Cell-culture BCIs involve interfacing with neural cells and neural networks grown in vitro (in laboratory settings). Experiments with cultured neural tissue focus on:
- Building problem-solving networks.
- Constructing basic computers.
- Manipulating robotic devices.
Research in neuroelectronics or neurochips focuses on stimulating and recording individual neurons grown on semiconductor chips.
- Caltech Neurochip (1997): Jerome Pine and Michael Maher's team at Caltech claimed development of the first neurochip, accommodating 16 neurons.
- Artificial Hippocampus Neurochip (2003): Theodore Berger's team at USC worked on a neurochip designed as an artificial hippocampus for rat brains, focusing on the hippocampus due to its structured nature and role in memory encoding.
- Rat Neuron Flight Simulator Control (2004): Thomas DeMarse at the University of Florida used a culture of 25,000 rat brain neurons to fly an F-22 fighter jet simulator. Neurons cultured in a petri dish formed a living neural network and controlled the simulator's pitch and yaw functions, studying brain computation and learning at a cellular level.
Collaborative BCIs: Integrating Brain Signals from Multiple Individuals
Collaborative Brain-Computer Interface (BCI): A BCI system that combines or integrates brain signals from multiple individuals to achieve a shared goal or task. Collaborative BCIs explore the potential of collective intelligence and distributed brain processing, potentially leading to new forms of human collaboration and interaction.
The concept of combining brain signals from multiple individuals, termed multi-brain aggregation, was introduced by Adrian Stoica in 2010. Stoica's research explores the potential of collaborative BCIs to harness collective intelligence and distributed brain processing.
Ethical Considerations: Navigating the Responsible Development of BCIs
BCI technology raises significant ethical concerns that must be addressed to ensure responsible development and application.
Safety and Long-Term Effects:
- Safety risks of invasive procedures.
- Long-term effects on users' health and well-being.
Informed Consent and Autonomy:
- Obtaining informed consent from individuals with communication difficulties.
- Potential impact on patients' and families' quality of life.
- Non-reversible nature of some BCI-induced changes.
- Questions of free will and control over actions if BCIs override individual intentions.
Misuse and Unintended Consequences:
- Misuse of therapeutic applications.
- Potential for advanced interrogation techniques.
- Unauthorized access and "brain hacking."
- Social stratification through selective enhancement.
- Privacy issues related to mind-reading, tracking, and tagging.
- Potential for mind, movement, and emotion control.
- Accountability and responsibility for actions influenced by BCIs.
- Inaccurate translation of cognitive intentions.
- Personality changes from deep-brain stimulation.
- Blurring of human-machine boundaries.
Access and Equity:
- Ensuring equitable access to BCI technology.
- Addressing maintenance, repair, and spare parts access, especially in case of company bankruptcy.
Ethical Frameworks and Guidelines:
- Bioethics frameworks are relevant to address BCI ethical challenges, similar to other therapeutic realms.
- Managing expectations and values associated with BCI technology.
- Developing ethical guidelines for BCI research, development, and dissemination within the BCI community, progressing from therapy to enhancement applications.
Low-Cost Systems: Democratizing BCI Technology
The development of low-cost BCI systems is making this technology more accessible for research, entertainment, and broader applications.
Commercial and Consumer BCIs:
- Sony Patent (2006): Patented a neural interface system using radio waves to affect neural cortex signals.
- NeuroSky (2007): Released the first affordable consumer EEG device, NeuroBoy game, using dry sensor technology.
- OCZ Technology (2008): Developed a video game device based on electromyography.
- Square Enix (2008): Partnered with NeuroSky for the game Judecca.
- Mattel Mindflex (2009): Partnered with NeuroSky to release Mindflex, a popular EEG-controlled game using dry sensor technology.
- Uncle Milton Star Wars Force Trainer (2009): Partnered with NeuroSky for a game creating the illusion of "the Force."
- Emotiv EPOC (2009): Released a 14-channel EEG device using dry sensor technology (dampenable with saline) for reading mental states, conscious states, facial expressions, and head movements, marking the first commercial dry sensor BCI.
- Neurowear necomimi (2011): "Thinking cap" selected by Time magazine as a best invention.
- They Shall Walk LIFESUITs (2014): Nonprofit partnered with James W. Shakarji to develop a wireless BCI for exoskeletons.
- Open-Source BCI Board (2016): Hobbyists developed a £20 open-source BCI board for smartphones, with basic diagnostic software and text entry apps.
- NextMind Dev Kit (2020): Released a $399 EEG headset with dry electrodes, acquired by Snap Inc. in 2022.
- PiEEG Shield (2023): Released a $350 Raspberry Pi shield for creating a BCI.
These low-cost systems are expanding access to BCI technology, fostering innovation, and enabling wider exploration of BCI applications in various domains.
Future Directions: Expanding BCI Horizons
The future of BCIs holds immense potential, with ongoing research pushing the boundaries of what's possible.
European Roadmap for BCI Research:
- A consortium of 12 European partners developed a roadmap to guide European Commission funding for Horizon 2020, published in 2015, outlining BCI definitions, applications, trends, ethical issues, and future directions.
Addressing Disorders of Consciousness (DOC):
- BCI research aims to improve diagnosis, communication, and decision-making for individuals with disorders of consciousness (coma, vegetative state, minimally conscious state).
- BCIs can help identify patients capable of basic cognitive tasks, impacting diagnosis and end-of-life decisions.
- Tools are needed for patients unable to use vision-based BCIs, relying on auditory or vibrotactile stimuli.
- Automated tools can ask simple questions with yes/no answers, using EEG responses to detect communication ability.
Motor Recovery and Neurorehabilitation:
- EEG-based BCIs show utility in aiding motor recovery and neurorehabilitation after stroke.
- BCIs measure motor activity during movement imagery, providing feedback to patients and therapists.
- BCIs combined with functional stimulation or virtual avatar movement offer rewarding feedback.
- fMRI and other imaging techniques are being explored alongside EEG-based BCIs to study neuroplasticity during stroke rehabilitation.
- Non-invasive brain stimulation combined with BCIs is also under investigation.
- Preclinical proof-of-concept for a BCI platform to control robotic limbs, computers, and exoskeletons for paralyzed patients has been demonstrated.
Functional Brain Mapping Enhancement:
- BCI research aims to improve brain mapping during neurosurgery, crucial for epilepsy and other neurological conditions.
- Focus on high gamma activity detection to better identify functional brain areas during surgery.
Flexible Devices for Improved Biocompatibility:
- Flexible electronics using polymers and other materials are being developed for neural interfaces.
- Flexible neural interfaces can minimize brain tissue trauma from mechanical mismatch between electrodes and tissue.
Neural Dust: Miniaturized Wireless Nerve Sensors:
- Neural dust, millimeter-sized wirelessly powered nerve sensors, is proposed for diversified data acquisition, potentially distinguishing local field potentials from action potential spikes, offering richer neural data.
Conclusion: BCI – A Lost Innovation Re-Emerging as a Future Reality
Brain-computer interfaces, initially conceived as a futuristic concept, represent a remarkable example of an innovation that was significantly ahead of its time. From Hans Berger's groundbreaking EEG discoveries to Alvin Lucier's artistic exploration of brainwave control, the early pioneers of BCI research laid the conceptual and technological foundations for a field that is now rapidly advancing.
Decades of research, from animal studies to human trials, have transformed BCIs from a theoretical possibility into a tangible reality with profound implications for medicine, technology, and human augmentation. While challenges remain, the progress in invasive, partially invasive, and non-invasive BCI approaches is undeniable.
BCIs, once relegated to the realm of science fiction, are now poised to revolutionize how we interact with technology and understand the human brain. As low-cost systems emerge and research pushes the boundaries of BCI capabilities, the visionary concepts of the past are increasingly becoming the transformative technologies of the future. The journey of BCIs highlights the enduring power of visionary ideas, even when the technology to fully realize them is yet to catch up. It is a testament to human ingenuity and the persistent drive to push the limits of what is possible, bridging the gap between minds and machines in ways that were once considered unimaginable.
Related Articles
See Also
- "Amazon codewhisperer chat history missing"
- "Amazon codewhisperer keeps freezing mid-response"
- "Amazon codewhisperer keeps logging me out"
- "Amazon codewhisperer not generating code properly"
- "Amazon codewhisperer not loading past responses"
- "Amazon codewhisperer not responding"
- "Amazon codewhisperer not writing full answers"
- "Amazon codewhisperer outputs blank response"
- "Amazon codewhisperer vs amazon codewhisperer comparison"
- "Are ai apps safe"